Welcome to the very first post of Art Science Millennial, a newsletter for non-techies navigating the world of tech! I know the struggle because I’m one of you.
When I think of automation, I picture a robotic arm whirling away tirelessly on a factory floor. Automation without a physical manifestation - say, a spreadsheet performing various calculations in a split second - rarely has the same evocative effect.
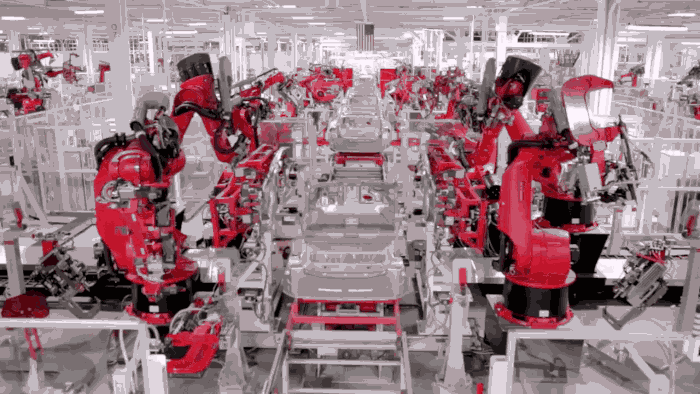
Human-free zone in a Tesla factory.
Perhaps that is why we associate the impact of automation with blue collar jobs more readily. For the longest time, I never thought too deeply about what automation meant for me as a writer. After all, writing is an act of self-expression and what could be more human than that? I suspect that many people who do creative work - including writers, photographers, designers - similarly feel little anxiety about the rise of the robots.
Yet while machines are still not able to replace humans in many aspects of creative work (and indeed, that day may never come), I believe that:
Automation largely driven by Artificial Intelligence (AI) is already having a profound impact on creative workers, and
That impact will only grow larger given the rapid nature of technological progress.
Consider the newsroom, a workplace where I spent seven years as a journalist. In 2019, The New York Times published an article, ominously headlined The Rise of the Robot Reporter, in which it wrote:
The program can dissect a financial report the moment it appears and spit out an immediate news story that includes the most pertinent facts and figures. And unlike business reporters, who find working on that kind of thing a snooze, it does so without complaint.
Untiring and accurate, Cyborg helps Bloomberg in its race against Reuters, its main rival in the field of quick-twitch business financial journalism, as well as giving it a fighting chance against a more recent player in the information race, hedge funds, which use artificial intelligence to serve their clients fresh facts.
And further on:
In addition to leaning on the software to generate minor league and college game stories, The A.P., like Bloomberg, has used it to beef up its coverage of company earnings reports. Since joining forces with Automated Insights, The A.P. has gone from producing 300 articles on earnings reports per quarter to 3,700.
In today’s hyper-competitive online news landscape, speed and volume are winners. More newsrooms will probably want in, particularly as the costs of adoption falls over time. What does this mean for flesh-and-blood reporters? The editors interviewed in the article emphasised they saw the tech as a tool that frees up time for reporters to engage in more meaningful work, investigating and finding their own stories:
“The work of journalism is creative, it’s about curiosity, it’s about storytelling, it’s about digging and holding governments accountable, it’s critical thinking, it’s judgment — and that is where we want our journalists spending their energy,” said Lisa Gibbs, the director of news partnerships for The A.P.
Indeed, when I was still in the newsroom, my colleagues and I weren’t exactly falling over ourselves to write these diary stories (so termed as they arrive on a fixed schedule). So there’s some truth in the words of these newsroom leaders. But there are implications that aren’t explored in the piece.
For one thing, such “boring” articles are usually the training ground of rookie reporters. They get drilled in writing speedily and accurately, the facts are easily checked, and close supervision is less needed. What will happen when all diary stories are written by robots? We may well discover that world-class journalists can still be nurtured without this rite of passage, but this is a conversation that needs to take place now, not when the technology has already become a widespread reality.
Like what you’re reading so far? Sign up so you don’t miss the next update of Art Science Millennial!
As computers get better at recognising images by the day, the work of photographers will also inevitably change.
Here’s a video of The New York Times’ chief data scientist speaking about automation in editing photos, between the 17:10 and 18:35 marks. (It’s worth watching the video in its entirety to get a fascinating peek at how The New York Times is using data science to drive journalism excellence and business growth.)
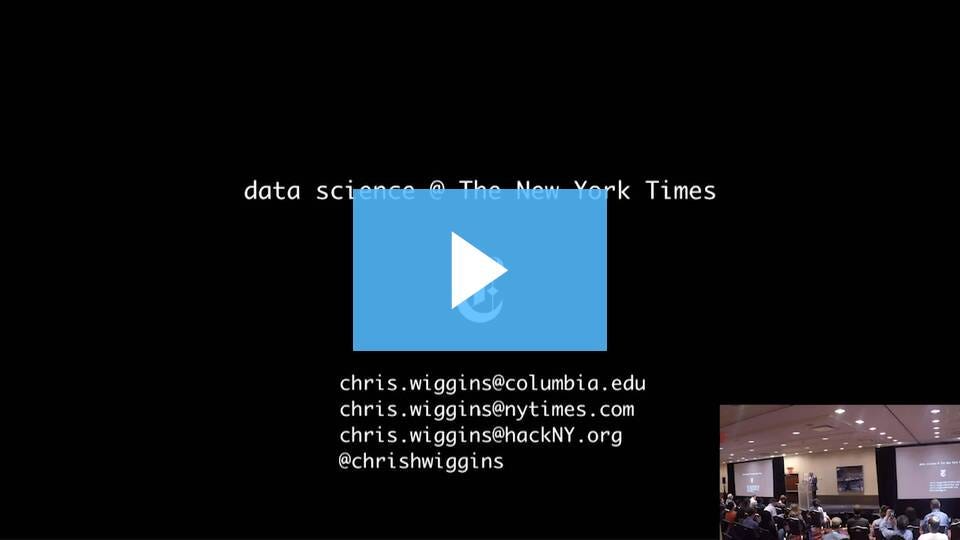
I’ve also produced the relevant transcript here:
Another problem The New York Times has is associated with dead trees, is putting ink onto dead trees. So when a photojournalist takes a picture and then sends it to the editors of The New York Times, which happens thousands of times a day, some of those pictures are going to end up becoming things that are in print. The file comes out of a camera or a phone or what have you, or a battery-free surveillance device built into the wall, who knows? Then a very careful and patient editor has to go through and re-balance all of the colour histograms, all the CMYK, until it comes out to exactly the right photo balance because otherwise you get just a black square when it eventually becomes ink and it goes to the printing press.
En route, in the form of exhaust, we have an awesome before and after dataset of before and after a patient editor did that and now we can basically give them a warm start. We can say: “Look, here’s the picture, here’s the file that came out of the photojournalist’s camera. Here’s our suggestion for how an infinitely patient editor is going to do it.” And using deep learning we can even go beyond that and we can say: “Here’s how editor number 12 is probably going to re-balance it,” versus, “Here’s…” You can actually learn different editor styles if you have enough data set.
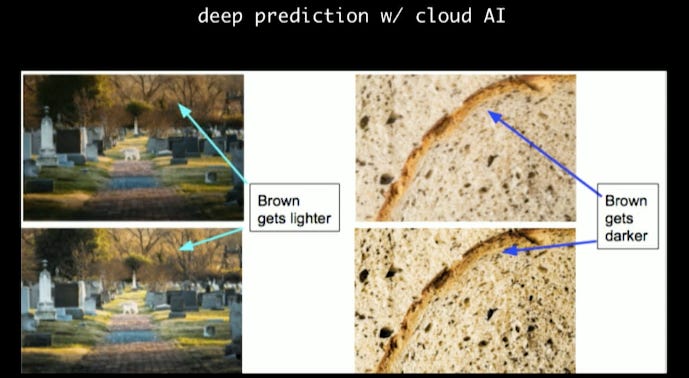
In a wide-ranging presentation, one of the topics discussed by The New York Times’ chief data scientist was automated photo editing.
In other words, given enough samples of the work of a number of photo editors, the deep learning model can not just replicate the work of a photo editor, it can replicate the work of a specific photo editor.
While it’s clear that The New York Times is using the tool to aid editors, not to replace them, the long-term effect may well be the need for fewer photo editors. As previously mentioned, speed matters in journalism. Traditionally, speed is achieved by boosting manpower. Today, it seems like speed can continue to increase even with a smaller team.
I raise these examples not as proof that creative work is imperilled by technology. Rather, creative workers should get savvy about technology and see opportunities, not threats. For instance, a freelance photographer juggling a wedding shoot every weekend will probably see the auto-photo editing tool as a god-send.
Machine learning (where machines learn to recognise things through examples instead of explicit instructions) is also enabling a whole new level of journalism stories such as:
How easy it is to build and deploy a facial recognition system using publicly available data, and
Identifying spy planes flying over the US.
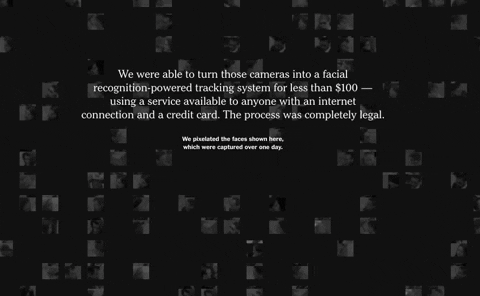
The New York Times demonstrates how easy it is to be a (legal) creep.
It’s quite a leap to expect all reporters to become experts in machine learning. But they must understand the principles underpinning this technology to be able to conceptualise such stories and work with data scientists to deliver them.
And as technology becomes ubiquitous in all aspects of life, it is not just tech reporters who have to know tech. As more companies strive to act like tech entities, even a business reporter writing about McDonald’s will have to know enough about data science to ask intelligent questions about its recommender system.
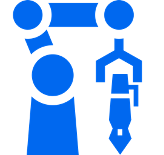
I chose a robotic arm holding a pen as the logo of this newsletter to remind myself that machines are now capable of mimicking certain aspects of creative work. And while it may not be a perfect impression, it’s only going to improve over time.
While I wrote about examples from journalism because that’s where most of my experience comes from, I believe the takeaways are just as applicable to other forms of creative work. We who chose creative careers (and who were probably “arts students” back in school) live in the same world as other people, and this is a world increasingly shaped by technology. We owe it to ourselves to learn more about tech’s impact on our lives.
In 2019, I put my media career on hold to attend a full-time data science boot camp. Eight months after that move, I finally found a job in data analytics at a start-up. In this newsletter, I’ll be writing once a week about the challenges in navigating the realm of tech as a non-techie, drawing from my experience switching from a creative career to tech work. Some of the topics I want to write about include:
How people who consider themselves analogue creatures can go about sparking a genuine and sustained curiosity about all things digital.
What are some of the pitfalls I fell into - and that you can avoid - while picking up tech skills.
And above all, why we should do away with the traditional arts/science divide and embrace an arts-science outlook in life.
It’s been an eventful journey so far. I hope you’ll come along for the rest of the ride.
Thank you for reading the very first post of Art Science Millennial! If you enjoyed this piece, sign up so you get subsequent updates in your inbox!
I loved reading this; if you are open to a collaboration, feel free to reach out to me at gabriel@inreallife.my (www.inreallife.my)