Is WP's Raeesah Khan the most disliked politician on social media in Singapore?
Blackbox Research’s sentiment analysis indicates so, but how that conclusion was reached is arguably more important
Welcome to Art Science Millennial, a newsletter for non-techies navigating the world of tech! I know the struggle because I’m one of you.
Was public opinion of the Workers’ Party’s Raeesah Khan significantly affected by the police report made against her and the subsequent scrutiny of her past social media posts?
That question seemed to have been emphatically answered when she went from candidate to MP-elect: Her team pulled off a rare victory in Sengkang GRC, only the second multi-seat ward to come under opposition control.
However, a study released last week by Blackbox Research, which is mentioned in several news reports, suggests that negative perceptions of Raeesah did take root. Based on social media data gathered during the 2020 general election campaign period, Blackbox produced the following infographic:
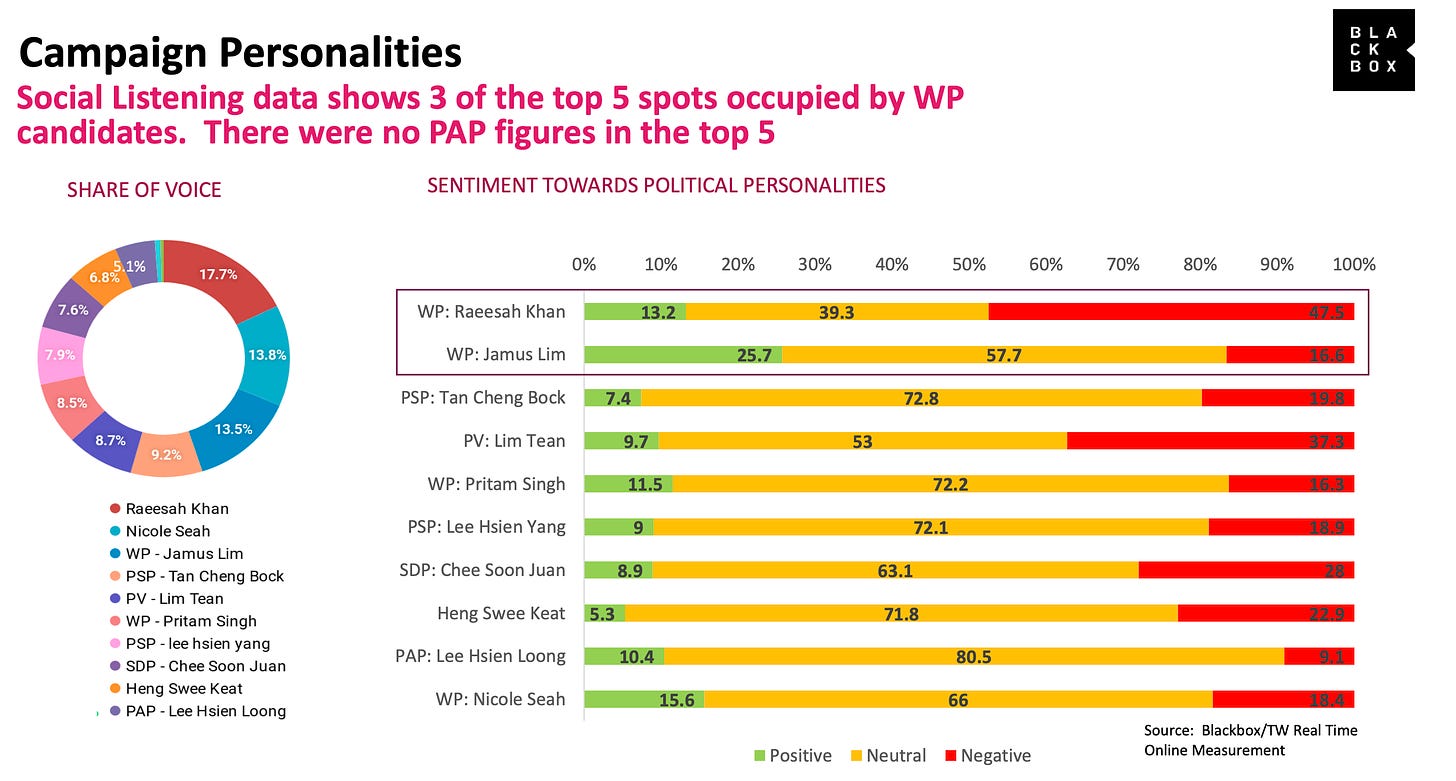
Page 18 of Blackbox’s “Singapore General Election 2020 Campaign Polling Summary”.
Raeesah was not just the most discussed politician, but also drew the most negative sentiment (even more than this election season’s chief provocateur Lim Tean).
So how was online sentiment gauged for this study?
To find out more, I reached out to Blackbox’s CEO David Black, who generously gave permission to use this segment of the study but understandably declined to go into specifics, citing the need to protect his company’s proprietary practices. Nonetheless, he confirmed that the results were derived from sentiment analysis, a tool that we should understand better given its growing usage and influence.
What is sentiment analysis?
Imagine you had an army of workers tirelessly reading every single social media post about a particular topic. A worker reads a post, decides whether that post expresses a positive or negative sentiment, then assigns a sentiment score between 1 (most positive) and -1 (most negative) to the post.
Replace the workers with an automated system and you get basic idea of how sentiment analysis is done. Existing systems are smart enough to recognise some context and online parlance, so if we consider the following tweets:
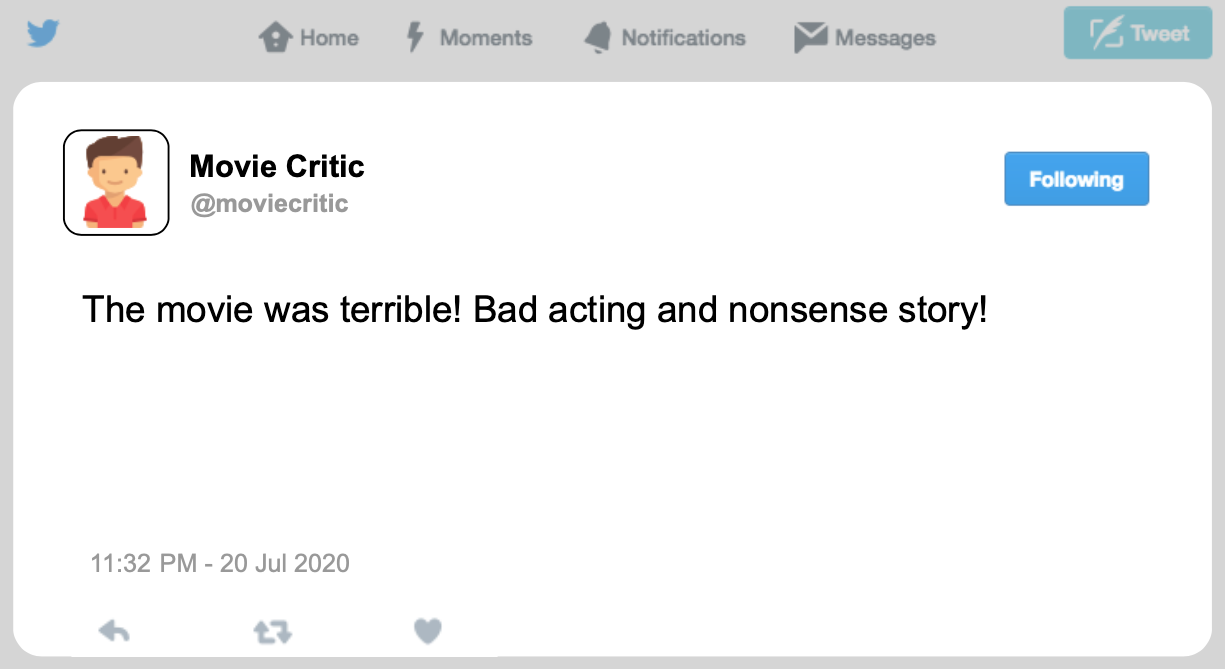
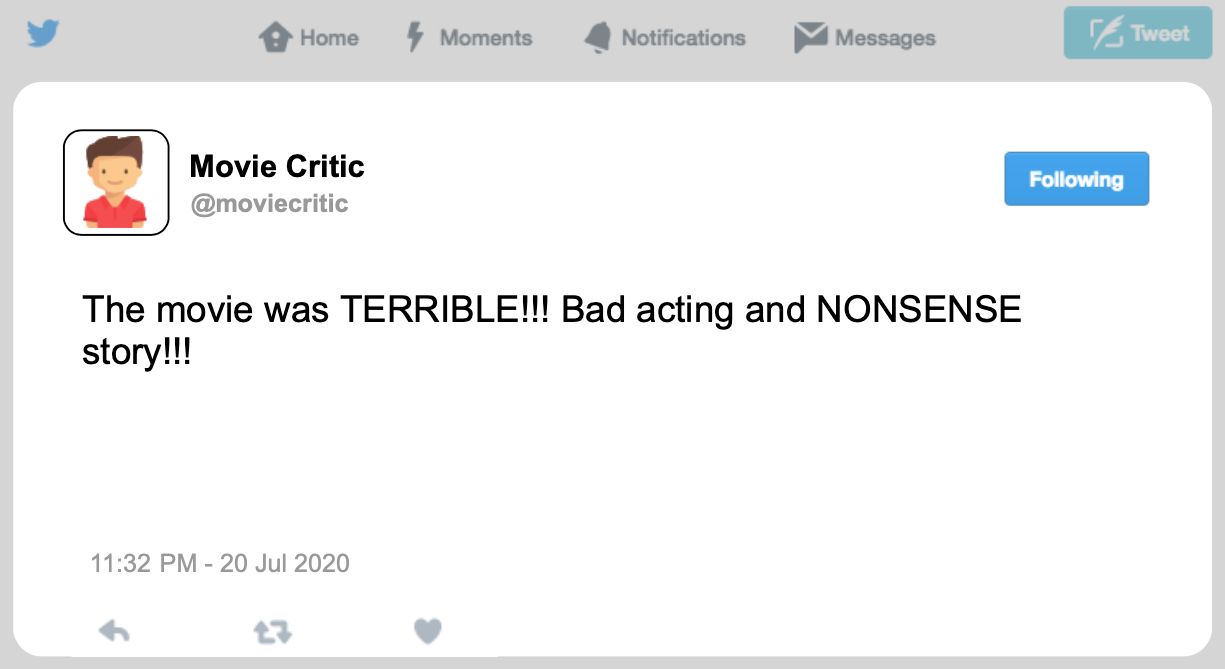
The second tweet will get a much lower sentiment score (perhaps -0.9) than the first (maybe just a -0.7) because the negative tilt is amplified by the liberal use of caps and exclamation marks. You can visit this link to enter your hypothetical tweets and test out one of the many sentiment analysis products on the market today.
Reading and rating thousands of messages within seconds is a great productivity boon, but is not without its imperfections. Sensing the tone of a post is a challenge and sarcasm is one of those human things that 10-year-olds can pull off effortlessly but which machines might fumble.
Companies that offer sentiment analysis services therefore emphasise their ability to correctly evaluate posts such as this example:
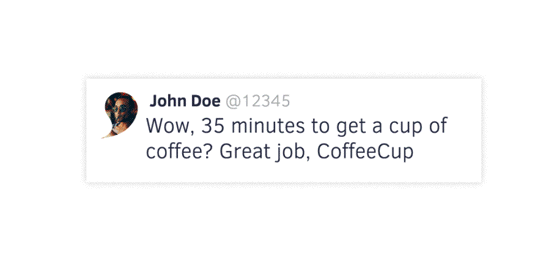
Source: Talkwalker, the social listening company that worked with Blackbox on the study.
And it’s not just reputation-conscious firms that are tapping on sentiment analysis. Financial information services such as Bloomberg and Refinitiv are selling sentiment analysis as yet another weapon in their arsenal to predict market movements.
The Singapore government has also gotten in on the act. In a 2013 speech, former head of the civil service Peter Ho — who retains senior roles in several government bodies — said:
Sentiment analysis can potentially help to improve public service and government policies by monitoring public opinion from behind the scenes to help policy-makers gauge the public’s pulse.
In Singapore, we are experimenting with sentiment analysis as part of the effort to “sense-make” and characterise online sentiments in the social media. Through such experiments, we are learning how social media data can help us better understand issues of concern to people, and the effect of policies on them.
The government’s use of sentiment analysis goes beyond social media. For instance, the Ministry of Trade and Industry created a new economic indicator by scoring sentiment of local newspaper articles “to obtain a higher-frequency and more real-time measure of economic sentiments”. This new benchmark was found to have a strong correlation with the traditional economic indicator of Gross Domestic Product growth, suggesting that it has similar usefulness as GDP in ascertaining the economy’s health.
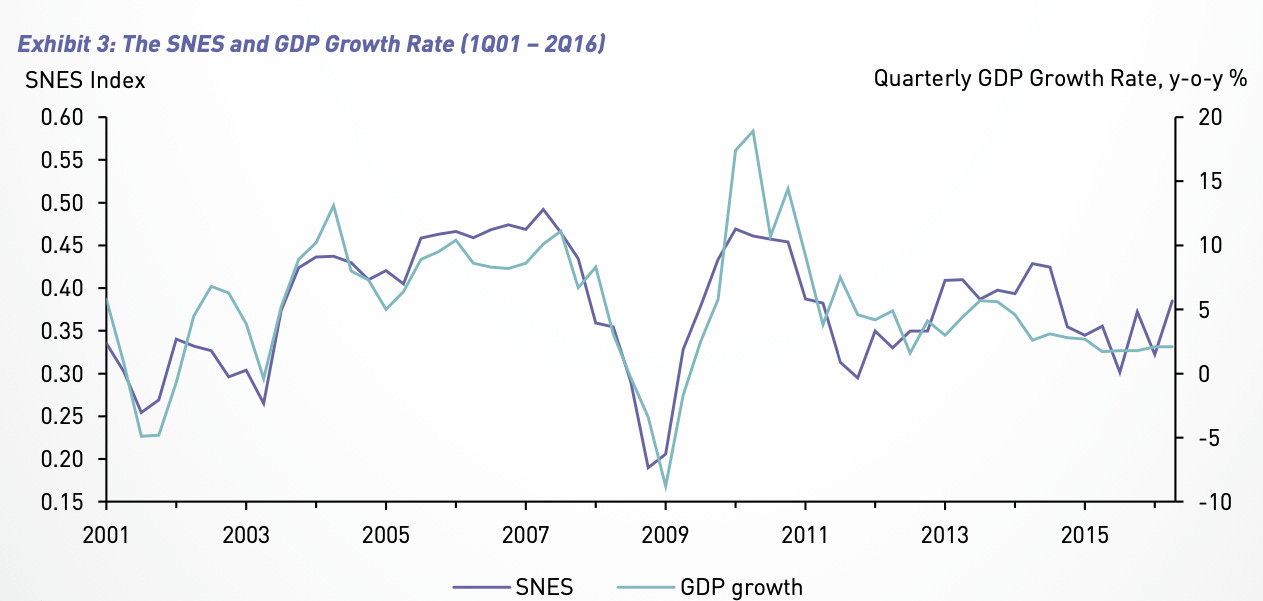
Source: Ministry of Trade and Industry. SNES, or the Singapore News Economic Sentiment Index, is derived from running sentiment analysis on economic-related articles published in local newspapers.
For those interested in exploring further, open source sentiment analysis tools typically require some coding, but the level of technical ability required is far from insurmountable. At the end of a 12-week data science bootcamp, I was able to modify an open source sentiment analyser and use it to score The Business Times’ articles, producing an economic indicator similar to the ministry’s research (the site is unfortunately not optimised for mobile and takes some time to load as it’s hosted for free).
Like what you’re reading so far? Sign up so you don’t miss the next update of Art Science Millennial!
Just how neutral is a neutral social media post?
Returning to the Blackbox sentiment analysis, we can see that sentiment is classified into three categories: negative, neutral, positive.
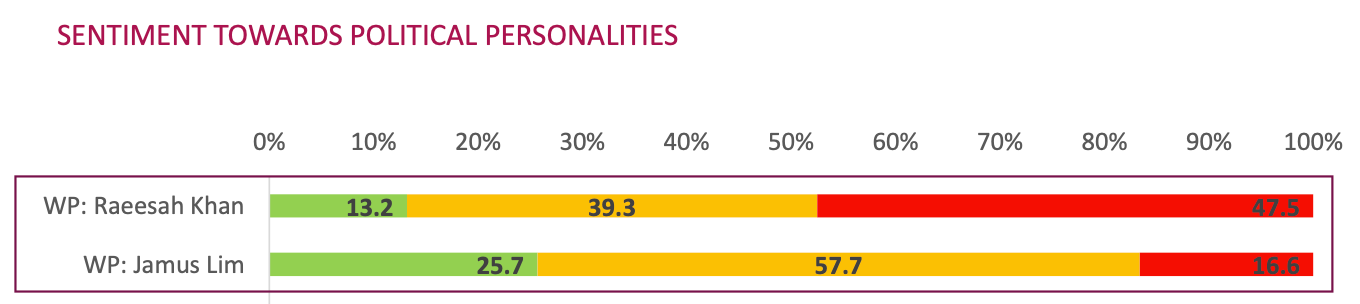
Green for positive, yellow for neutral, red for negative.
Recall that each social media post is scored on a scale of -1 (most negative) to 1 (most positive). To create the three sentiment categories, you set thresholds — say, any post scoring above 0.2 is considered positive while any post below -0.2 is considered negative. Anything in between would be neutral.
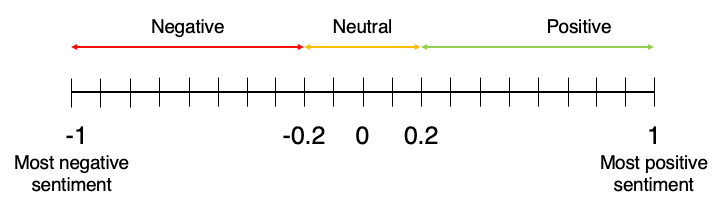
Posts scoring more than -0.2 and less than 0.2 fall into the neutral zone.
If you wanted to be stricter in terms of what should be considered positive or negative, simply widen the neutral territory. A post would have to show higher intensity of sentiment — moving its score closer to either ends of the spectrum — to be not considered neutral.
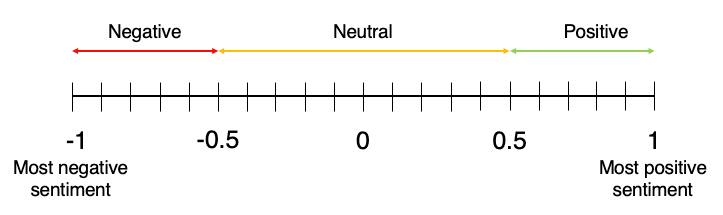
A more conservative approach: posts would have to score more than 0.5 to be considered positive and less than -0.5 to be considered negative.
As you can tell, some discernment is needed to determine the best thresholds. For example, a reasonable way might be to find a bunch of tweets that sound neutral and use their sentiment scores as the benchmark. So if the scores for those neutral sounding tweets are roughly between -0.3 and 0.3, use those numbers as the threshold. This is a seemingly logical approach, except that someone else reading the same tweets might not find them neutral at all.
What is the yardstick for authenticity?
Another relevant factor is the source of a social media post. Does the high negative sentiment captured for Raeesah reflect genuine online backlash, or is the data picking up some form of concerted attack by partisan groups?
Research on astroturfing and the related problem of fake reviews has been going on for years and I think it is safe to assume some form of detection has been built into Blackbox’s sentiment analysis algorithms. Systems in general can even be adjusted to discount posts from certain sources or even disregard them altogether.
Who then should we box out of the equation? There are, of course, straightforward cases of fake accounts — bots with just three friends, users who signed up just a week ago. Beyond that, we have to ponder the bigger question of authenticity, which is harder to resolve than at first glance.
Consider a situation where a group of people purposefully drops a large number of negative comments, skewing the results of the sentiment analysis. Such a coordinated effort may seem like inauthentic behaviour, but the volume could also just be a sign of the magnitude of feelings towards the subject. One way to deal with this is to ignore subsequent messages from a user that crosses a certain number of posts on a topic, which again necessitates some judgment.
Despite the unavoidable subjectivity involved, the issue of authenticity must be grappled with. Perhaps organisations can — without revealing technical, competitive information — describe the broad strokes they take in sentiment analysis, what forms of behaviour is considered inauthentic, and the guidelines to keep the data free from such influence. This is akin to how opinion polls disclose sample sizes and sample gathering methods, so as to enable people to better evaluate for themselves the study’s robustness.
A complement, not a replacement
Ultimately, we are probably not at the stage where social media sentiment analysis can stand alone. Blackbox’s study collected other data through more traditional means such as opinion polls and focus groups. It not only assessed the partys’ online share of voice, but also their presence on the ground.
In my short exchange with David Black, he said information from the digital realm complements — rather than replaces — that from the physical world, and will continue to do so for some time to come:
If you compare the People’s Action Party’s online share of voice and offline visibility they are remarkably similar. Mix and match seems to be a good method for now until artificial intelligence advances and attitudes and emotions expressed in digital fora can be better analysed. We’re still in the Stone Age on that.
I’d love to know what you think of this newsletter and what you’d like me to write about. You can reach me at zi.liang.chong@gmail.com or by leaving a comment if you’re reading this on the Art Science Millennial website. If you enjoyed this piece, sign up so you get subsequent updates in your inbox!